Table of Contents
Key Takeaway
AI is transforming the finance sector by enhancing accuracy, efficiency, and decision-making capabilities. From predicting market trends to detecting fraud, AI tools are becoming indispensable in financial analysis.
While AI offers significant advantages, such as improved predictive accuracy and real-time insights, it also presents challenges like data quality dependency, high implementation costs, and ethical concerns such as algorithmic bias.
The future of finance is increasingly intertwined with AI. As the technology evolves, financial institutions must address these challenges and ethical considerations to fully harness AI’s potential and maintain trust in the industry.
The financial sector has always been at the forefront of adopting new technologies, and artificial intelligence (AI) is no exception. AI is revolutionizing the finance industry by enhancing various processes and enabling more accurate decision-making. The role of AI in finance is multifaceted, ranging from automating routine tasks to providing deep insights through advanced data analysis. Financial institutions are increasingly relying on AI to manage large volumes of data, predict market trends, and improve customer service.
The global AI in finance market is projected to grow from $8.3 billion in 2023 to $27.3 billion by 2027, with a CAGR of 33.8% during the forecast period.
AI tools are becoming indispensable in financial analysis due to their ability to process vast amounts of data quickly and accurately. These tools leverage machine learning algorithms and data analytics to identify patterns, forecast future trends, and uncover insights that would be impossible for humans to detect manually. By doing so, AI enhances the accuracy, speed, and reliability of financial analysis.
For instance, AI-driven algorithms can analyze historical data to predict stock prices or assess the creditworthiness of loan applicants. This not only speeds up the decision-making process but also minimizes the risk of human error. Additionally, AI tools can adapt and learn from new data, continuously improving their performance over time.
Overview of AI in Financial Analysis
The Role of AI in Finance
AI is rapidly becoming an integral part of the finance sector. Financial institutions are increasingly adopting AI to automate routine tasks, manage large data sets, and gain deeper insights into market trends. This technology helps in making more informed and faster decisions, enhancing efficiency and productivity. Whether it’s predicting stock prices or improving customer service, AI is reshaping how financial services operate.
Importance of AI Tools in Financial Analysis
AI tools are revolutionizing financial analysis by significantly enhancing its accuracy, speed, and reliability. These tools employ machine learning algorithms and advanced data analytics to uncover patterns and trends that would be difficult for humans to detect. For example, AI can quickly analyze historical data to forecast market movements or assess credit risk, minimizing human error and speeding up decision-making processes. As AI systems learn and adapt over time, their performance continues to improve, making financial analysis more precise and dependable.
AI Tools for Financial Analysis
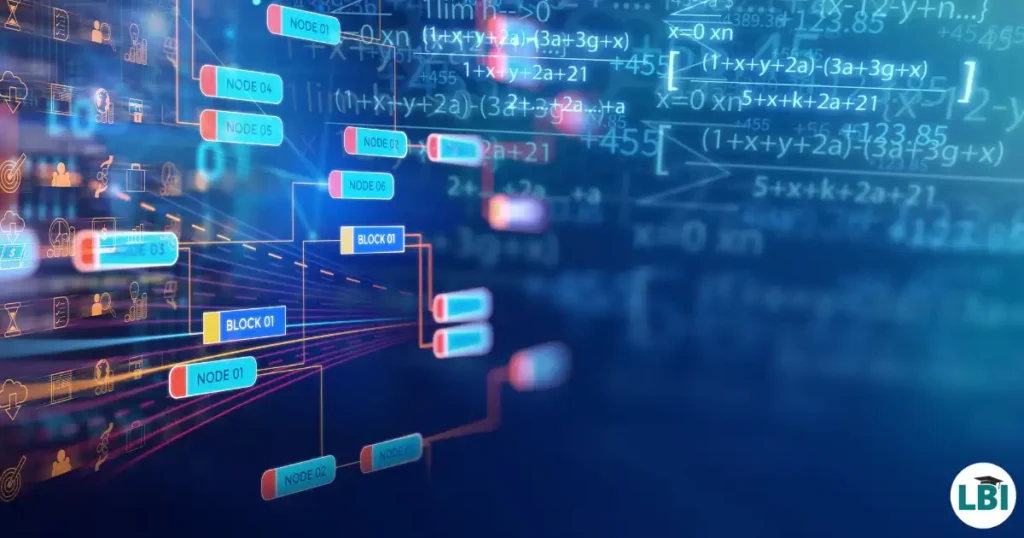
Machine Learning Algorithms
Machine learning algorithms are at the heart of AI tools used in financial analysis. They bring a range of benefits to the table, but there are also some drawbacks to consider.
Benefits:
- Enhanced Predictive Accuracy: Machine learning models can analyze vast amounts of data, leading to more precise financial forecasts. This ability to predict market trends and movements with higher accuracy helps investors and financial analysts make better-informed decisions.
- Pattern Recognition: These algorithms excel at identifying complex patterns and correlations in data. This capability is crucial for improving investment strategies and risk management decisions, as it allows analysts to uncover insights that might not be evident through traditional analysis methods.
- Automation of Repetitive Tasks: By automating data analysis, machine learning frees up analysts to focus on more strategic planning and decision-making. Routine tasks such as data entry, sorting, and basic analysis can be handled by AI, increasing overall efficiency.
Drawbacks:
- Data Quality Dependency: The accuracy of machine learning models heavily relies on the quality and quantity of the input data. Poor or limited data can lead to incorrect predictions and analyses, undermining the reliability of the insights generated.
- Complexity: Developing and maintaining machine learning models requires significant expertise and resources. The complexity of these models means that financial institutions must invest in skilled personnel and advanced technology to fully leverage their benefits.
- Overfitting Risk: There is a risk that models might perform exceptionally well on historical data but fail to generalize in real-world scenarios if overfitting occurs. Overfitting happens when a model is too closely tailored to the training data, capturing noise rather than the underlying patterns, which can lead to poor performance on new, unseen data.
Machine learning algorithms offer substantial advantages in terms of predictive accuracy, pattern recognition, and automation, they also come with challenges related to data quality, complexity, and the risk of overfitting. Properly addressing these drawbacks is essential for maximizing the effectiveness of AI in financial analysis.
- A report by McKinsey indicates that AI-driven predictive analytics can improve forecasting accuracy by up to 20-30%, leading to better financial decision-making and risk management.
- According to a PwC study, automating data analysis with AI could reduce the time spent on data management by up to 70%, allowing analysts to focus on more strategic tasks.
Natural Language Processing (NLP)
Natural Language Processing (NLP) is another powerful AI tool used in financial analysis. It processes and understands human language, offering several benefits and some drawbacks.
Benefits:
- Sentiment Analysis: NLP helps analyze market sentiment by processing news articles, financial reports, and social media posts. This provides valuable insights into market trends and investor sentiment, helping analysts gauge the mood of the market and make more informed decisions.
- Automation of Report Analysis: NLP can quickly sift through large volumes of textual data, such as earnings reports and regulatory filings, to extract relevant information. This automation saves time and reduces the manual effort required to analyze these documents, allowing analysts to focus on higher-level analysis.
- Improved Decision-Making: By understanding language and context, NLP tools can assist in making more informed investment decisions. They can interpret complex documents, identify key themes, and provide summaries, thereby enhancing the decision-making process.
Drawbacks:
- Language Nuances: NLP may struggle with understanding context, sarcasm, or slang, which can lead to potential misinterpretation of sentiment or data. These subtleties in language can pose challenges for NLP systems, especially in analyzing informal or nuanced text.
- Data Privacy Concerns: Processing large volumes of textual data, especially from social media, raises concerns about data privacy and ethics. Ensuring that data is handled responsibly and in compliance with privacy regulations is a significant challenge.
- High Implementation Costs: Developing and integrating NLP systems can be costly, particularly for small and medium-sized firms. The initial setup, ongoing maintenance, and need for specialized expertise can make NLP a significant investment.
NLP offers substantial advantages in sentiment analysis, report automation, and decision-making, but it also faces challenges related to language nuances, data privacy, and implementation costs. Balancing these factors is crucial for successfully leveraging NLP in financial analysis.
- Research shows that NLP-powered sentiment analysis can increase the accuracy of market predictions by approximately 10-15%, helping investors better understand market movements.
- A survey by Deloitte found that 42% of financial institutions are already using or planning to implement NLP tools in their operations.
AI-Powered Financial Software
AI-powered financial software is becoming increasingly popular due to its ability to provide comprehensive data analysis and real-time insights. However, like any technology, it also has its benefits and drawbacks.
List of AI-powered financial tools:
- Upmetrics
- Function: Financial modeling and forecasting
- Description: Upmetrics helps startups and small business owners create investment-ready business plans, financial budgets, statements, and visual reports. It automates manual and repetitive tasks, making financial modeling more accurate and efficient.
- FinChat.io
- Function: Investment research
- Description: FinChat.io is a generative AI tool for investment research. It reduces the time spent on data aggregation, visualization, and summaries. It provides detailed breakdowns of financial metrics, company insights, macroeconomic indicators, and market forecasts.
- Truewind.ai
- Function: Accounting
- Description: Truewind.ai automates accounting processes, making them more efficient and accurate. It helps with bookkeeping, financial reporting, and compliance.
- Mint
- Function: Personal finance management
- Description: Mint is a personal finance tool that helps users manage their budgets, track expenses, and monitor their financial health. It uses AI to provide personalized financial advice and insights.
- Datarails
- Function: Financial planning and analysis (FP&A)
- Description: Datarails is an FP&A tool that automates data consolidation, reporting, and analysis. It helps finance teams make data-driven decisions by providing real-time insights and forecasts.
- Planful
- Function: Financial planning and analysis (FP&A)
- Description: Planful is an AI-powered FP&A platform that streamlines financial planning, budgeting, and forecasting. It enhances collaboration and provides actionable insights to improve financial performance.
- SigFig
- Function: Portfolio management
- Description: SigFig uses AI to help financial advisors with portfolio optimization, risk assessment, and trend prediction. It provides data-driven insights to enhance investment strategies.
- Datamaran
- Function: Risk management
- Description: Datamaran uses AI to analyze and monitor risk factors in real-time. It helps financial institutions identify and mitigate potential risks, ensuring compliance and stability.
- PulseFolio
- Function: Investment analysis
- Description: PulseFolio leverages AI to provide in-depth investment analysis and recommendations. It helps investors make informed decisions by analyzing market trends and financial data.
Benefits:
- Comprehensive Data Analysis: AI-powered software can analyze multiple data sources simultaneously, offering a holistic view of financial markets. This comprehensive approach allows for more accurate and informed financial decisions, as it considers a wide range of factors and data points.
- Real-Time Insights: These tools provide real-time analysis and alerts, enabling quicker decision-making. Financial analysts and investors can react swiftly to market changes, capitalize on opportunities, and mitigate risks effectively.
- User-Friendly Interfaces: Many AI-powered financial platforms are designed with intuitive interfaces, making them accessible to users with varying levels of expertise. This democratizes access to advanced financial analysis tools, allowing both seasoned professionals and novices to benefit from AI technology.
Drawbacks:
- Reliance on Software Providers: Financial firms may become dependent on specific AI software vendors, limiting their flexibility. Switching providers or customizing software solutions can be challenging and costly, especially if the firm has deeply integrated a particular platform into its operations.
- Cost of Adoption: Advanced AI software often comes with high licensing fees and implementation costs. For smaller firms, the financial burden of adopting such technology can be prohibitive, limiting their ability to compete with larger institutions that can afford these investments.
- Security Risks: AI-powered software can be vulnerable to cyberattacks, potentially leading to data breaches or financial loss. Ensuring robust cybersecurity measures and staying updated with the latest security protocols is essential to protect sensitive financial data and maintain trust.
AI-powered financial software offers significant advantages in terms of comprehensive data analysis, real-time insights, and user-friendly interfaces. However, challenges such as reliance on specific software providers, high adoption costs, and security risks must be carefully managed to fully leverage the potential of this technology in financial analysis.
- Financial firms using AI-powered software have reported a 25% improvement in decision-making speed due to real-time data processing and analysis.
- The use of AI in financial trading is growing rapidly, with 45% of hedge funds utilizing AI-driven strategies, contributing to improved performance and risk management.
AI in Fraud Detection
AI has become an essential tool in the fight against fraud within the financial sector. By leveraging advanced algorithms and real-time data analysis, AI systems provide several key benefits, though they also come with certain drawbacks.
Benefits:
- Proactive Risk Management: AI tools can detect fraudulent activities in real-time, allowing firms to mitigate risks before they escalate. This proactive approach helps prevent financial losses and protects both the institution and its customers from fraudulent schemes.
- Scalability: AI systems can monitor vast numbers of transactions across multiple platforms, far beyond human capability. This scalability ensures that even high volumes of transaction data are scrutinized for potential fraud, providing a comprehensive security net.
- Continuous Learning: AI fraud detection systems improve over time by learning from new data and evolving fraud tactics. As these systems are exposed to more data, they become better at identifying subtle patterns and sophisticated fraud attempts, enhancing their effectiveness.
Drawbacks:
- False Positives: AI systems may sometimes flag legitimate transactions as fraudulent, leading to unnecessary disruptions. These false positives can inconvenience customers and create additional workload for fraud investigation teams tasked with verifying the flagged transactions.
- High Setup and Maintenance Costs: Implementing and maintaining AI fraud detection systems can be expensive. The initial investment in technology, coupled with ongoing maintenance and updates, can be a significant financial burden for some institutions.
- Privacy Concerns: The extensive monitoring required for effective fraud detection may raise concerns about customer privacy. Ensuring that AI systems comply with privacy regulations and handle sensitive data responsibly is crucial to maintaining customer trust.
In conclusion, AI in fraud detection offers significant benefits in terms of proactive risk management, scalability, and continuous learning. However, challenges such as false positives, high setup and maintenance costs, and privacy concerns need to be carefully addressed to maximize the effectiveness of AI in combating financial fraud.
- AI systems can reduce fraud detection times by up to 90%, with some banks reporting a 50% reduction in fraud-related losses after implementing AI-driven detection systems.
- The global financial industry could save up to $15 billion annually by 2025 through the implementation of AI fraud detection systems.
Real-World Examples of AI in Financial Analysis
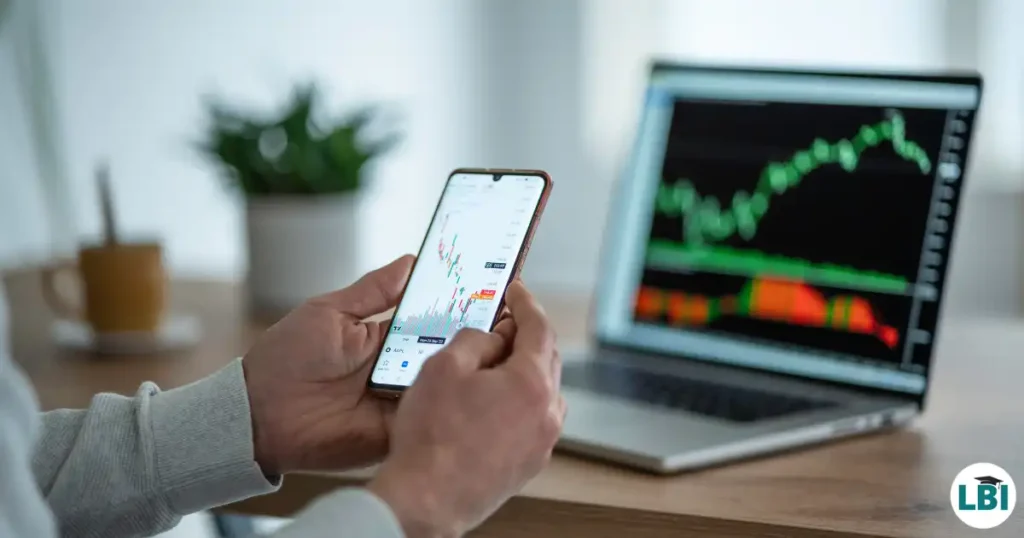
Leading Financial Institutions Using AI
Example 1: JPMorgan Chase’s use of COIN (Contract Intelligence) JPMorgan Chase has developed and deployed a software called COIN (Contract Intelligence) that automates the document review process for certain types of contracts. This AI-powered system uses machine learning and image recognition to analyze and categorize clauses in credit agreements. By doing so, COIN has significantly reduced the time required for document review, completing tasks in seconds that previously took lawyers over 360,000 man-hours annually. This not only improves efficiency but also enhances accuracy, reducing the risk of human error.
Example 2: BlackRock’s Aladdin platform BlackRock’s Aladdin platform is an advanced AI-driven system used for risk management and portfolio management. Aladdin unifies the investment management process through a common data language, providing a comprehensive view of portfolios across public and private markets. The platform leverages sophisticated risk analytics, machine learning, and big data to help investors make informed decisions, identify opportunities, and manage risks effectively. Aladdin’s capabilities have made it a cornerstone in the investment management industry, managing assets worth trillions of dollars.
Success Stories
Story 1: How a hedge fund improved its trading strategies using AI-driven predictive analytics
Hedge funds have increasingly turned to AI-driven predictive analytics to enhance their trading strategies. For instance, Point72 Asset Management has launched a new AI-focused hedge fund that leverages advanced AI technologies to analyze market data and identify trading opportunities. By using AI algorithms, the fund can process vast amounts of data at high speeds, enabling it to detect price patterns, market trends, and arbitrage opportunities that are often invisible to human traders. This approach has not only improved the accuracy and efficiency of their trading strategies but also increased their overall profitability.
Story 2: Banks that significantly reduced fraud through AI-based detection systems
Banks have also benefited from AI-based detection systems to combat fraud. For example, many financial institutions have adopted AI and machine learning technologies to proactively identify and mitigate fraudulent activities. These AI systems analyze vast datasets to detect unusual patterns and anomalies in real-time, allowing banks to respond promptly to potential fraud threats. By shifting from reactive to proactive measures, banks have significantly reduced fraud losses and enhanced the security of financial transactions. This strategic adoption of AI not only protects customers but also secures the integrity of the banking system.
Challenges and Ethical Considerations
As AI continues to transform the financial sector, it brings along several challenges and ethical considerations that must be addressed.
Challenges:
- Data Quality: The effectiveness of AI systems heavily relies on the quality of the data they process. Inaccurate, incomplete, or biased data can lead to incorrect predictions and decisions. Ensuring high-quality, clean data is crucial for the success of AI applications in finance.
- Integration Complexity: Integrating AI systems with existing financial infrastructure can be complex and time-consuming. Financial institutions often have legacy systems that are not designed to work with modern AI technologies, making seamless integration a significant challenge.
- Need for Skilled Professionals: Developing, implementing, and maintaining AI systems requires highly skilled professionals with expertise in data science, machine learning, and finance. There is a growing demand for such talent, and financial institutions must invest in training and hiring to keep up with technological advancements.
Ethical Concerns:
- Algorithmic Bias: AI systems can inadvertently perpetuate biases present in the data they are trained on. This can lead to unfair outcomes, such as biased credit scoring or discriminatory lending practices. Ensuring that AI models are fair and unbiased is a critical ethical concern.
- Data Privacy Issues: AI systems often require access to vast amounts of personal and sensitive data. This raises concerns about data privacy and the potential for misuse of information. Financial institutions must adhere to strict data protection regulations and implement robust security measures to safeguard customer data.
- Importance of Human Oversight: While AI can automate many tasks, human oversight remains essential to ensure ethical and accurate decision-making. Relying solely on AI can lead to unintended consequences, and it is important to have human experts review and validate AI-driven outcomes.
Conclusion
AI is revolutionizing the financial sector by enhancing accuracy, efficiency, and decision-making capabilities. From machine learning algorithms that predict market trends to NLP tools that analyze sentiment, AI is transforming how financial institutions operate. Leading firms like JPMorgan Chase and BlackRock are leveraging AI to streamline processes and manage risks more effectively.
However, the adoption of AI comes with challenges such as data quality issues, integration complexities, and the need for skilled professionals. Ethical concerns like algorithmic bias, data privacy, and the necessity of human oversight must also be addressed to ensure the responsible use of AI.
Balancing innovation with ethical practices will enable the financial sector to fully benefit from AI’s potential while maintaining trust and fairness.
Businesses are leveraging AI for various tasks other than finance. To learn more also read: AI for Business: Key Trends and Insights
FAQ
How is AI improving financial analysis?
AI improves financial analysis by automating data processing, enhancing predictive accuracy, and providing real-time insights, allowing financial analysts to make more informed and faster decisions.
What are the main benefits of using machine learning algorithms in finance?
Machine learning algorithms offer enhanced predictive accuracy, superior pattern recognition, and automation of repetitive tasks, which collectively improve investment strategies and risk management.
How does Natural Language Processing (NLP) benefit financial institutions?
NLP benefits financial institutions by enabling sentiment analysis, automating the analysis of large volumes of textual data, and improving decision-making through better understanding of language and context.
What are some drawbacks of using AI in financial fraud detection?
Drawbacks include the potential for false positives, high setup and maintenance costs, and privacy concerns due to extensive monitoring of transactions.
Why is data quality important for AI systems in finance?
Data quality is crucial because AI systems rely on accurate and comprehensive data to make reliable predictions and decisions. Poor data quality can lead to incorrect outcomes and undermine the system’s effectiveness.
How are leading financial institutions using AI technology?
Leading financial institutions like JPMorgan Chase use AI for legal document analysis with COIN, while BlackRock employs its Aladdin platform for risk management and portfolio optimization, showcasing diverse applications of AI in finance.
What ethical concerns are associated with AI in finance?
Ethical concerns include algorithmic bias, data privacy issues, and the necessity of human oversight to ensure fair and accurate decision-making processes.
What challenges do financial institutions face when integrating AI?
Challenges include ensuring data quality, dealing with integration complexities with existing systems, and the need for skilled professionals with expertise in both AI and finance.
How does AI-powered financial software benefit users?
AI-powered financial software provides comprehensive data analysis, real-time insights, and user-friendly interfaces, making advanced financial tools accessible to users of varying expertise levels.
What measures can be taken to address the ethical concerns of AI in finance?
Measures include rigorous testing for algorithmic bias, implementing robust data privacy protocols, and maintaining strong human oversight to validate AI-driven decisions and ensure ethical practices.